PEP 550 – 执行上下文
- 作者:
- Yury Selivanov <yury at edgedb.com>, Elvis Pranskevichus <elvis at edgedb.com>
- 状态:
- 已撤回
- 类型:
- 标准轨迹
- 创建:
- 2017年8月11日
- Python 版本:
- 3.7
- 历史记录:
- 2017年8月11日,2017年8月15日,2017年8月18日,2017年8月25日,2017年9月1日
摘要
此 PEP 添加了一种新的通用机制,用于确保在无序执行(例如在 Python 生成器和协程中)的上下文中一致访问非本地状态。
线程本地存储,例如 threading.local()
,对于在同一操作系统线程中并发执行的程序来说是不够的。此 PEP 提出了解决此问题的方案。
PEP 状态
由于其广度以及某些方面缺乏普遍共识,此 PEP 已被撤回,并由一个更简单的 PEP 567 取代,该 PEP 已被接受并包含在 Python 3.7 中。
PEP 567实现了相同的核心思想,但将 ContextVar 支持限制在异步任务中,同时保持生成器行为不变。后者可能会在未来的 PEP 中重新审视。
基本原理
在 Python 中出现异步编程之前,程序使用操作系统线程来实现并发。线程特定状态的需求通过 threading.local()
及其 C-API 等效项 PyThreadState_GetDict()
解决。
一些常见依赖线程本地存储 (TLS) 的示例
- 像十进制上下文、
numpy.errstate
和warnings.catch_warnings
这样的上下文管理器。 - 与请求相关的数据,例如 Web 应用程序中的安全令牌和请求数据,
gettext
等的语言上下文。 - 大型代码库中的分析、跟踪和日志记录。
不幸的是,TLS 对于在单个线程中并发执行的程序效果不佳。Python 生成器是最简单的并发程序示例。考虑以下情况
def fractions(precision, x, y):
with decimal.localcontext() as ctx:
ctx.prec = precision
yield Decimal(x) / Decimal(y)
yield Decimal(x) / Decimal(y ** 2)
g1 = fractions(precision=2, x=1, y=3)
g2 = fractions(precision=6, x=2, y=3)
items = list(zip(g1, g2))
items
的直观预期值是
[(Decimal('0.33'), Decimal('0.666667')),
(Decimal('0.11'), Decimal('0.222222'))]
令人惊讶的是,实际结果是
[(Decimal('0.33'), Decimal('0.666667')),
(Decimal('0.111111'), Decimal('0.222222'))]
这是因为隐式十进制上下文存储为线程本地,因此 fractions()
生成器的并发迭代会破坏状态。具体来说,对于十进制,目前唯一的解决方法是对所有算术运算使用显式上下文方法调用 [28]。可以说,这破坏了重载运算符的用处,并使即使是简单的公式也难以阅读和编写。
协程是另一类 Python 代码,其中 TLS 的不可靠性是一个重大问题。
异步代码中 TLS 的不足导致了临时解决方案的激增,这些解决方案的范围有限,并且不支持所有必需的用例。
目前现状是,任何依赖 TLS 的库(包括标准库)在异步代码或与生成器一起使用时都可能出现问题(例如 [3])。
一些支持协程或生成器的语言建议将上下文手动作为参数传递给每个函数,例如 [1]。但是,这种方法对 Python 的用途有限,Python 中存在一个庞大的生态系统,该生态系统构建为使用类似 TLS 的上下文。此外,像 decimal
或 numpy
这样的库在重载运算符实现中隐式地依赖于上下文。
.NET 运行时支持 async/await,它有一个针对此问题的通用解决方案,称为 ExecutionContext
(参见 [2])。
目标
此 PEP 的目标是提供一个更可靠的 threading.local()
替代方案,该方案
- 提供机制和 API 来修复协程和生成器的非本地状态问题;
- 为同步代码实现类似 TLS 的语义,以便像
decimal
和numpy
这样的用户可以切换到新机制,而不会有破坏向后兼容性的风险; - 对现有代码或将使用新机制的代码(包括 C 扩展)没有或几乎没有性能影响。
高级规范
此 PEP 的完整规范分为三个部分
- 高级规范(本节):整体解决方案的描述。我们展示了它如何应用于用户代码中的生成器和协程,而无需深入了解实现细节。
- 详细规范:新概念、API 和标准库相关更改的完整描述。
- 实现细节:用于实现此 PEP 的数据结构和算法的描述和分析,以及对 CPython 的必要更改。
出于本节的目的,我们将执行上下文定义为一个不透明的非本地状态容器,它允许在并发执行环境中一致地访问其内容。
上下文变量是一个表示执行上下文中的值的 object。调用 contextvars.ContextVar(name)
会创建一个新的上下文变量 object。上下文变量 object 有三个方法
get()
:返回当前执行上下文中的变量值;set(value)
:设置当前执行上下文中的变量值;delete()
:可用于恢复变量状态,其用途和语义在 设置和恢复上下文变量 中解释。
常规单线程代码
在不涉及生成器或协程的常规单线程代码中,上下文变量的行为类似于全局变量
var = contextvars.ContextVar('var')
def sub():
assert var.get() == 'main'
var.set('sub')
def main():
var.set('main')
sub()
assert var.get() == 'sub'
多线程代码
在多线程代码中,上下文变量的行为类似于线程本地变量
var = contextvars.ContextVar('var')
def sub():
assert var.get() is None # The execution context is empty
# for each new thread.
var.set('sub')
def main():
var.set('main')
thread = threading.Thread(target=sub)
thread.start()
thread.join()
assert var.get() == 'main'
生成器
与常规函数调用不同,生成器可以协作地将其执行控制权让给调用方。此外,生成器不控制在何处执行将在其产生后继续。它可能从任意代码位置恢复。
出于这些原因,生成器的最不令人惊讶的行为如下
- 对上下文变量的更改始终是本地的,在外部上下文中不可见,但在生成器调用的代码中可见;
- 一旦在生成器中设置,上下文变量保证在迭代之间不会更改;
- 外部上下文(其中生成器正在被迭代)中对上下文变量的更改对生成器可见,除非这些变量也在生成器内部被修改。
让我们回顾一下
var1 = contextvars.ContextVar('var1')
var2 = contextvars.ContextVar('var2')
def gen():
var1.set('gen')
assert var1.get() == 'gen'
assert var2.get() == 'main'
yield 1
# Modification to var1 in main() is shielded by
# gen()'s local modification.
assert var1.get() == 'gen'
# But modifications to var2 are visible
assert var2.get() == 'main modified'
yield 2
def main():
g = gen()
var1.set('main')
var2.set('main')
next(g)
# Modification of var1 in gen() is not visible.
assert var1.get() == 'main'
var1.set('main modified')
var2.set('main modified')
next(g)
现在,让我们重新审视 基本原理 部分中的十进制精度示例,并了解执行上下文如何改善这种情况
import decimal
# create a new context var
decimal_ctx = contextvars.ContextVar('decimal context')
# Pre-PEP 550 Decimal relies on TLS for its context.
# For illustration purposes, we monkey-patch the decimal
# context functions to use the execution context.
# A real working fix would need to properly update the
# C implementation as well.
def patched_setcontext(context):
decimal_ctx.set(context)
def patched_getcontext():
ctx = decimal_ctx.get()
if ctx is None:
ctx = decimal.Context()
decimal_ctx.set(ctx)
return ctx
decimal.setcontext = patched_setcontext
decimal.getcontext = patched_getcontext
def fractions(precision, x, y):
with decimal.localcontext() as ctx:
ctx.prec = precision
yield MyDecimal(x) / MyDecimal(y)
yield MyDecimal(x) / MyDecimal(y ** 2)
g1 = fractions(precision=2, x=1, y=3)
g2 = fractions(precision=6, x=2, y=3)
items = list(zip(g1, g2))
items
的值是
[(Decimal('0.33'), Decimal('0.666667')),
(Decimal('0.11'), Decimal('0.222222'))]
这与预期结果相符。
协程和异步任务
与生成器一样,协程可以产生并重新获得控制权。与生成器的主要区别在于,协程不会产生到直接调用方。相反,整个协程调用堆栈(通过 await
链式连接的协程)切换到另一个协程调用堆栈。在这方面,await
一个协程在概念上类似于常规函数调用,而协程链(或“任务”,例如 asyncio.Task
)在概念上类似于线程。
从这种相似性中,我们得出结论,协程中的上下文变量应该表现得像“任务本地变量”
- 对协程中上下文变量的更改对等待它的协程可见;
- 在等待之前在调用方进行的上下文变量更改对被等待的协程可见;
- 在一个任务中进行的上下文变量更改在其他任务中不可见;
- 由其他任务产生的任务从父任务继承执行上下文,但在子任务产生之后在父任务中对上下文变量进行的任何更改都不可见。
最后一点显示的行为与操作系统线程不同。操作系统线程默认不继承执行上下文。有两个原因:常见的用法意图和向后兼容性。
任务继承上下文而线程不继承上下文的主要原因是常见的用法意图。任务通常用于与产生任务的代码逻辑相关的相对较短的运行操作(例如在 asyncio 中使用超时运行协程)。另一方面,操作系统线程通常用于长时间运行的、逻辑上独立的代码。
关于向后兼容性,我们希望执行上下文的行为类似于 threading.local()
。这样,库就可以开始使用执行上下文代替 TLS,从而降低破坏与现有代码的兼容性的风险。
让我们回顾一些示例来说明我们刚刚定义的语义。
单个任务中的上下文变量传播
import asyncio
var = contextvars.ContextVar('var')
async def main():
var.set('main')
await sub()
# The effect of sub() is visible.
assert var.get() == 'sub'
async def sub():
assert var.get() == 'main'
var.set('sub')
assert var.get() == 'sub'
loop = asyncio.get_event_loop()
loop.run_until_complete(main())
任务之间的上下文变量传播
import asyncio
var = contextvars.ContextVar('var')
async def main():
var.set('main')
loop.create_task(sub()) # schedules asynchronous execution
# of sub().
assert var.get() == 'main'
var.set('main changed')
async def sub():
# Sleeping will make sub() run after
# "var" is modified in main().
await asyncio.sleep(1)
# The value of "var" is inherited from main(), but any
# changes to "var" made in main() after the task
# was created are *not* visible.
assert var.get() == 'main'
# This change is local to sub() and will not be visible
# to other tasks, including main().
var.set('sub')
loop = asyncio.get_event_loop()
loop.run_until_complete(main())
如上所示,对执行上下文的更改仅限于任务本地,并且任务在创建时获取执行上下文的快照。
有一种狭义的边缘情况会导致意外行为。考虑以下示例,其中我们在嵌套协程中修改上下文变量
async def sub(var_value):
await asyncio.sleep(1)
var.set(var_value)
async def main():
var.set('main')
# waiting for sub() directly
await sub('sub-1')
# var change is visible
assert var.get() == 'sub-1'
# waiting for sub() with a timeout;
await asyncio.wait_for(sub('sub-2'), timeout=2)
# wait_for() creates an implicit task, which isolates
# context changes, which means that the below assertion
# will fail.
assert var.get() == 'sub-2' # AssertionError!
但是,依赖上下文更改泄漏到调用者最终是一种不好的模式。出于这个原因,上述示例中显示的行为不被认为是一个主要问题,可以通过适当的文档来解决。
详细规范
从概念上讲,执行上下文(EC)是逻辑上下文的堆栈。每个 Python 线程始终只有一个活动的 EC。
逻辑上下文(LC)是上下文变量与其在该特定 LC 中的值的映射。
上下文变量是表示执行上下文中值的对象。通过调用 contextvars.ContextVar(name: str)
创建新的上下文变量对象。所需 name
参数的值不用于 EC 机制,但可用于调试和内省。
上下文变量对象具有以下方法和属性
name
:传递给ContextVar()
的值。get(*, topmost=False, default=None)
,如果 topmost 为False
(默认值),则从上到下遍历执行上下文,直到找到变量值。如果 topmost 为True
,则返回最顶层逻辑上下文中变量的值。如果未找到变量值,则返回 default 的值。set(value)
:设置最顶层逻辑上下文中变量的值。delete()
:从最顶层逻辑上下文中删除变量。在将逻辑上下文恢复到set()
调用之前的状态时很有用,例如,在上下文管理器中,有关更多信息,请参阅 设置和恢复上下文变量。
生成器
创建时,每个生成器对象在其 __logical_context__
属性中存储一个空的逻辑上下文对象。此逻辑上下文在每次生成器迭代开始时推送到执行上下文中,并在结束时弹出
var1 = contextvars.ContextVar('var1')
var2 = contextvars.ContextVar('var2')
def gen():
var1.set('var1-gen')
var2.set('var2-gen')
# EC = [
# outer_LC(),
# gen_LC({var1: 'var1-gen', var2: 'var2-gen'})
# ]
n = nested_gen() # nested_gen_LC is created
next(n)
# EC = [
# outer_LC(),
# gen_LC({var1: 'var1-gen', var2: 'var2-gen'})
# ]
var1.set('var1-gen-mod')
var2.set('var2-gen-mod')
# EC = [
# outer_LC(),
# gen_LC({var1: 'var1-gen-mod', var2: 'var2-gen-mod'})
# ]
next(n)
def nested_gen():
# EC = [
# outer_LC(),
# gen_LC({var1: 'var1-gen', var2: 'var2-gen'}),
# nested_gen_LC()
# ]
assert var1.get() == 'var1-gen'
assert var2.get() == 'var2-gen'
var1.set('var1-nested-gen')
# EC = [
# outer_LC(),
# gen_LC({var1: 'var1-gen', var2: 'var2-gen'}),
# nested_gen_LC({var1: 'var1-nested-gen'})
# ]
yield
# EC = [
# outer_LC(),
# gen_LC({var1: 'var1-gen-mod', var2: 'var2-gen-mod'}),
# nested_gen_LC({var1: 'var1-nested-gen'})
# ]
assert var1.get() == 'var1-nested-gen'
assert var2.get() == 'var2-gen-mod'
yield
# EC = [outer_LC()]
g = gen() # gen_LC is created for the generator object `g`
list(g)
# EC = [outer_LC()]
上面的代码片段显示了生成器整个生命周期中执行上下文堆栈的状态。
contextlib.contextmanager
contextlib.contextmanager()
装饰器可用于将生成器转换为上下文管理器。可以这样定义临时修改上下文变量值的上下文管理器
var = contextvars.ContextVar('var')
@contextlib.contextmanager
def var_context(value):
original_value = var.get()
try:
var.set(value)
yield
finally:
var.set(original_value)
不幸的是,这不会立即起作用,因为对 var
变量的修改包含在 var_context()
生成器中,因此在 with
块内不可见
def func():
# EC = [{}, {}]
with var_context(10):
# EC becomes [{}, {}, {var: 10}] in the
# *precision_context()* generator,
# but here the EC is still [{}, {}]
assert var.get() == 10 # AssertionError!
解决此问题的办法是将生成器的 __logical_context__
属性设置为 None
。这将导致生成器避免修改执行上下文堆栈。
我们修改 contextlib.contextmanager()
装饰器以将 genobj.__logical_context__
设置为 None
以生成行为良好的上下文管理器
def func():
# EC = [{}, {}]
with var_context(10):
# EC = [{}, {var: 10}]
assert var.get() == 10
# EC becomes [{}, {var: None}]
枚举上下文变量
ExecutionContext.vars()
方法返回一个 ContextVar
对象列表,这些对象在执行上下文中具有值。此方法主要用于内省和日志记录。
协程
在 CPython 中,协程与生成器共享实现。不同之处在于,在协程中,__logical_context__
默认为 None
。这会影响 async def
协程和旧式基于生成器的协程(用 @types.coroutine
装饰的生成器)。
异步生成器
异步生成器中的执行上下文语义与普通生成器的语义没有区别。
asyncio
asyncio
使用 Loop.call_soon
、Loop.call_later
和 Loop.call_at
来调度函数的异步执行。asyncio.Task
使用 call_soon()
运行包装的协程。
我们修改 Loop.call_{at,later,soon}
以接受新的可选 execution_context 关键字参数,该参数默认为当前执行上下文的副本
def call_soon(self, callback, *args, execution_context=None):
if execution_context is None:
execution_context = contextvars.get_execution_context()
# ... some time later
contextvars.run_with_execution_context(
execution_context, callback, args)
contextvars.get_execution_context()
函数返回当前执行上下文的浅拷贝。这里的浅拷贝是指这样的新执行上下文
- 副本中的查找提供与原始执行上下文相同的结果,并且
- 原始执行上下文中的任何更改都不会影响副本,并且
- 对副本的任何更改都不会影响原始执行上下文。
以下任一满足复制要求
- 一个带有逻辑上下文浅拷贝的新堆栈;
- 一个带有单个压缩逻辑上下文的新堆栈。
contextvars.run_with_execution_context(ec, func, *args, **kwargs)
函数使用 ec 作为执行上下文运行 func(*args, **kwargs)
。该函数执行以下步骤
- 将 ec 设置为当前线程中的当前执行上下文堆栈。
- 将一个空逻辑上下文推送到堆栈中。
- 运行
func(*args, **kwargs)
。 - 从堆栈中弹出逻辑上下文。
- 恢复原始执行上下文堆栈。
- 返回或引发
func()
结果。
这些步骤确保 func 无法修改 ec,这使得 run_with_execution_context()
幂等。
asyncio.Task
的修改如下
class Task:
def __init__(self, coro):
...
# Get the current execution context snapshot.
self._exec_context = contextvars.get_execution_context()
# Create an empty Logical Context that will be
# used by coroutines run in the task.
coro.__logical_context__ = contextvars.LogicalContext()
self._loop.call_soon(
self._step,
execution_context=self._exec_context)
def _step(self, exc=None):
...
self._loop.call_soon(
self._step,
execution_context=self._exec_context)
...
生成器转换为迭代器
任何 Python 生成器都可以表示为等效的迭代器。像 Cython 这样的编译器依赖于这个公理。关于执行上下文,这样的迭代器应该表现得与它表示的生成器相同。
这意味着需要一个 Python API 来创建新的逻辑上下文并在给定的逻辑上下文中运行代码。
contextvars.LogicalContext()
函数创建一个新的空逻辑上下文。
contextvars.run_with_logical_context(lc, func, *args, **kwargs)
函数可用于在指定的逻辑上下文中运行函数。lc 可能会因调用而修改。
contextvars.run_with_logical_context()
函数执行以下步骤
- 将 lc 推送到当前执行上下文堆栈。
- 运行
func(*args, **kwargs)
。 - 从执行上下文堆栈中弹出 lc。
- 返回或引发
func()
结果。
通过使用 LogicalContext()
和 run_with_logical_context()
,我们可以像这样复制生成器行为
class Generator:
def __init__(self):
self.logical_context = contextvars.LogicalContext()
def __iter__(self):
return self
def __next__(self):
return contextvars.run_with_logical_context(
self.logical_context, self._next_impl)
def _next_impl(self):
# Actual __next__ implementation.
...
让我们看看如何将此模式应用于示例生成器
# create a new context variable
var = contextvars.ContextVar('var')
def gen_series(n):
var.set(10)
for i in range(1, n):
yield var.get() * i
# gen_series is equivalent to the following iterator:
class CompiledGenSeries:
# This class is what the `gen_series()` generator can
# be transformed to by a compiler like Cython.
def __init__(self, n):
# Create a new empty logical context,
# like the generators do.
self.logical_context = contextvars.LogicalContext()
# Initialize the generator in its LC.
# Otherwise `var.set(10)` in the `_init` method
# would leak.
contextvars.run_with_logical_context(
self.logical_context, self._init, n)
def _init(self, n):
self.i = 1
self.n = n
var.set(10)
def __iter__(self):
return self
def __next__(self):
# Run the actual implementation of __next__ in our LC.
return contextvars.run_with_logical_context(
self.logical_context, self._next_impl)
def _next_impl(self):
if self.i == self.n:
raise StopIteration
result = var.get() * self.i
self.i += 1
return result
对于手写的迭代器,这种上下文管理方法通常是不必要的,并且更容易直接在 __next__
中设置和恢复上下文变量
class MyIterator:
# ...
def __next__(self):
old_val = var.get()
try:
var.set(new_val)
# ...
finally:
var.set(old_val)
实现
执行上下文实现为逻辑上下文的不可变链接列表,其中每个逻辑上下文都是一个不可变的弱键映射。指向当前活动执行上下文的指针存储在操作系统线程状态中
+-----------------+
| | ec
| PyThreadState +-------------+
| | |
+-----------------+ |
|
ec_node ec_node ec_node v
+------+------+ +------+------+ +------+------+
| NULL | lc |<----| prev | lc |<----| prev | lc |
+------+--+---+ +------+--+---+ +------+--+---+
| | |
LC v LC v LC v
+-------------+ +-------------+ +-------------+
| var1: obj1 | | EMPTY | | var1: obj4 |
| var2: obj2 | +-------------+ +-------------+
| var3: obj3 |
+-------------+
选择不可变映射的不可变列表作为基本数据结构的动机是需要有效地实现 contextvars.get_execution_context()
,该函数将被异步任务和回调频繁使用。当 EC 不可变时,get_execution_context()
可以简单地通过引用复制当前执行上下文
def get_execution_context(self):
return PyThreadState_Get().ec
让我们回顾所有可能的上下文修改场景
- 调用
ContextVariable.set()
方法def ContextVar_set(self, val): # See a more complete set() definition # in the `Context Variables` section. tstate = PyThreadState_Get() top_ec_node = tstate.ec top_lc = top_ec_node.lc new_top_lc = top_lc.set(self, val) tstate.ec = ec_node( prev=top_ec_node.prev, lc=new_top_lc)
- 调用
contextvars.run_with_logical_context()
,在这种情况下,传递的逻辑上下文对象将附加到执行上下文中def run_with_logical_context(lc, func, *args, **kwargs): tstate = PyThreadState_Get() old_top_ec_node = tstate.ec new_top_ec_node = ec_node(prev=old_top_ec_node, lc=lc) try: tstate.ec = new_top_ec_node return func(*args, **kwargs) finally: tstate.ec = old_top_ec_node
- 调用
contextvars.run_with_execution_context()
,在这种情况下,当前执行上下文将设置为传递的执行上下文,并将一个新的空逻辑上下文附加到其中def run_with_execution_context(ec, func, *args, **kwargs): tstate = PyThreadState_Get() old_top_ec_node = tstate.ec new_lc = contextvars.LogicalContext() new_top_ec_node = ec_node(prev=ec, lc=new_lc) try: tstate.ec = new_top_ec_node return func(*args, **kwargs) finally: tstate.ec = old_top_ec_node
- 在
genobj
生成器上调用genobj.send()
、genobj.throw()
、genobj.close()
中的任何一个,在这种情况下,记录在genobj
中的逻辑上下文将被推送到堆栈中PyGen_New(PyGenObject *gen): if (gen.gi_code.co_flags & (CO_COROUTINE | CO_ITERABLE_COROUTINE)): # gen is an 'async def' coroutine, or a generator # decorated with @types.coroutine. gen.__logical_context__ = None else: # Non-coroutine generator gen.__logical_context__ = contextvars.LogicalContext() gen_send(PyGenObject *gen, ...): tstate = PyThreadState_Get() if gen.__logical_context__ is not None: old_top_ec_node = tstate.ec new_top_ec_node = ec_node( prev=old_top_ec_node, lc=gen.__logical_context__) try: tstate.ec = new_top_ec_node return _gen_send_impl(gen, ...) finally: gen.__logical_context__ = tstate.ec.lc tstate.ec = old_top_ec_node else: return _gen_send_impl(gen, ...)
- 协程和异步生成器与生成器共享实现,上述更改也适用于它们。
在某些情况下,可能需要压缩 EC 以限制链的大小。例如,考虑以下极端情况
async def repeat(coro, delay):
await coro()
await asyncio.sleep(delay)
loop.create_task(repeat(coro, delay))
async def ping():
print('ping')
loop = asyncio.get_event_loop()
loop.create_task(repeat(ping, 1))
loop.run_forever()
在上面的代码中,只要调用 repeat()
,EC 链就会增长。每个新任务都会调用 contextvars.run_with_execution_context()
,这会将新的逻辑上下文附加到链中。为了防止无限增长,contextvars.get_execution_context()
检查链是否长于预定的最大值,如果是,则将链压缩为单个 LC
def get_execution_context():
tstate = PyThreadState_Get()
if tstate.ec_len > EC_LEN_MAX:
squashed_lc = contextvars.LogicalContext()
ec_node = tstate.ec
while ec_node:
# The LC.merge() method does not replace
# existing keys.
squashed_lc = squashed_lc.merge(ec_node.lc)
ec_node = ec_node.prev
return ec_node(prev=NULL, lc=squashed_lc)
else:
return tstate.ec
逻辑上下文
逻辑上下文是不可变的弱键映射,它相对于垃圾回收具有以下属性
ContextVar
对象仅从应用程序代码中被强引用,而不是从任何执行上下文机制或它们指向的值中被强引用。这意味着没有可能延长其生命周期超过必要的引用循环,或者阻止 GC 收集它们。- 放入执行上下文中的值保证在该线程中存在
ContextVar
密钥引用它们时保持活动状态。 - 如果
ContextVar
被垃圾回收,则其所有值都将从所有上下文中删除,如果需要,允许它们被 GC 回收。 - 如果 OS 线程已结束执行,则其线程状态将与执行上下文一起被清理,清理该线程中与所有上下文变量绑定的所有值。
如前所述,我们需要 contextvars.get_execution_context()
无论执行上下文的大小如何,都能始终保持快速,因此逻辑上下文必须是不可变的映射。
选择使用dict
作为底层实现并非最优方案,因为LC.set()
会导致dict.copy()
操作,这是一个O(N)复杂度的操作,其中N是LC中项目的数量。
get_execution_context()
在压缩EC时,是一个O(M)复杂度的操作,其中M是EC中所有上下文变量值的总数。
因此,我们选择哈希数组映射Trie(HAMT)作为逻辑上下文的底层实现,而不是dict
。(Scala和Clojure使用HAMT来实现高性能的不可变集合[5],[6]。)
使用HAMT,.set()
变为O(log N)复杂度的操作,并且get_execution_context()
的压缩操作由于HAMT的结构共享,平均效率更高。
请参阅附录:HAMT性能分析,以获取关于HAMT与dict
性能对比的更详细分析。
上下文变量
ContextVar.get()
和ContextVar.set()
方法的实现如下(伪代码)
class ContextVar:
def get(self, *, default=None, topmost=False):
tstate = PyThreadState_Get()
ec_node = tstate.ec
while ec_node:
if self in ec_node.lc:
return ec_node.lc[self]
if topmost:
break
ec_node = ec_node.prev
return default
def set(self, value):
tstate = PyThreadState_Get()
top_ec_node = tstate.ec
if top_ec_node is not None:
top_lc = top_ec_node.lc
new_top_lc = top_lc.set(self, value)
tstate.ec = ec_node(
prev=top_ec_node.prev,
lc=new_top_lc)
else:
# First ContextVar.set() in this OS thread.
top_lc = contextvars.LogicalContext()
new_top_lc = top_lc.set(self, value)
tstate.ec = ec_node(
prev=NULL,
lc=new_top_lc)
def delete(self):
tstate = PyThreadState_Get()
top_ec_node = tstate.ec
if top_ec_node is None:
raise LookupError
top_lc = top_ec_node.lc
if self not in top_lc:
raise LookupError
new_top_lc = top_lc.delete(self)
tstate.ec = ec_node(
prev=top_ec_node.prev,
lc=new_top_lc)
为了在性能敏感的代码路径(例如numpy
和decimal
中)实现高效访问,我们在ContextVar.get()
中缓存查找结果,使其在命中缓存时成为O(1)复杂度的操作。缓存键由以下内容组成:
- 新的
uint64_t PyThreadState->unique_id
,这是一个全局唯一的线程状态标识符。它由新的uint64_t PyInterpreterState->ts_counter
计算得出,该计数器在创建新的线程状态时递增。 - 新的
uint64_t PyThreadState->stack_version
,这是一个线程特定的计数器,在将非空逻辑上下文推入堆栈或从堆栈弹出时递增。 uint64_t ContextVar->version
计数器,在任何操作系统线程中任何逻辑上下文中更改上下文变量值时递增。
缓存的实现如下:
class ContextVar:
def set(self, value):
... # implementation
self.version += 1
def get(self, *, default=None, topmost=False):
if topmost:
return self._get_uncached(
default=default, topmost=topmost)
tstate = PyThreadState_Get()
if (self.last_tstate_id == tstate.unique_id and
self.last_stack_ver == tstate.stack_version and
self.last_version == self.version):
return self.last_value
value = self._get_uncached(default=default)
self.last_value = value # borrowed ref
self.last_tstate_id = tstate.unique_id
self.last_stack_version = tstate.stack_version
self.last_version = self.version
return value
请注意,last_value
是一个借用的引用。我们假设如果版本检查正常,则值对象将保持存活。这允许正确地垃圾回收上下文变量的值。
这种通用的缓存方法类似于decimal
当前的C实现中用于缓存当前十进制上下文的做法,并且具有相似的性能特征。
性能注意事项
基于此PEP先前修订版本的参考实现的测试表明,生成器微基准测试的性能下降了1-2%,而在宏基准测试中没有明显的差异。
非生成器和非异步代码的性能不受此PEP的影响。
新API摘要
Python
此PEP引入了以下新的Python API:
- 新的
contextvars.ContextVar(name: str='...')
类,其实例具有以下特性:- 只读
.name
属性; .get()
方法,返回当前执行上下文中的变量值;.set()
方法,设置当前逻辑上下文中的变量值;.delete()
方法,从当前逻辑上下文中删除变量的值。
- 只读
- 新的
contextvars.ExecutionContext()
类,表示一个执行上下文。 - 新的
contextvars.LogicalContext()
类,表示一个逻辑上下文。 - 新的
contextvars.get_execution_context()
函数,返回一个ExecutionContext
实例,表示当前执行上下文的副本。 contextvars.run_with_execution_context(ec: ExecutionContext, func, *args, **kwargs)
函数,使用提供的执行上下文运行func。contextvars.run_with_logical_context(lc: LogicalContext, func, *args, **kwargs)
函数,使用提供的逻辑上下文在当前执行上下文的顶部运行func。
C API
PyContextVar * PyContext_NewVar(char *desc)
:创建一个PyContextVar
对象。PyObject * PyContext_GetValue(PyContextVar *, int topmost)
:返回当前执行上下文中的变量值。int PyContext_SetValue(PyContextVar *, PyObject *)
:设置当前逻辑上下文中的变量值。int PyContext_DelValue(PyContextVar *)
:从当前逻辑上下文中删除变量的值。PyLogicalContext * PyLogicalContext_New()
:创建一个新的空PyLogicalContext
。PyExecutionContext * PyExecutionContext_New()
:创建一个新的空PyExecutionContext
。PyExecutionContext * PyExecutionContext_Get()
:返回当前执行上下文。int PyContext_SetCurrent( PyExecutionContext *, PyLogicalContext *)
:将传递的EC对象设置为活动线程状态的当前执行上下文,以及/或者将传递的LC对象设置为当前逻辑上下文。
设计考虑
“yield from”应该泄漏上下文更改吗?
不会。有人可能会争辩说yield from
在语义上等同于调用函数,并且应该泄漏上下文更改。但是,不可能同时满足以下条件:
next(gen)
不会泄漏gen
中发生的上下文更改;yield from gen
会泄漏gen
中发生的上下文更改。
原因是yield from
可以与部分迭代的生成器一起使用,该生成器已经存在局部上下文更改。
var = contextvars.ContextVar('var')
def gen():
for i in range(10):
var.set('gen')
yield i
def outer_gen():
var.set('outer_gen')
g = gen()
yield next(g)
# Changes not visible during partial iteration,
# the goal of this PEP:
assert var.get() == 'outer_gen'
yield from g
assert var.get() == 'outer_gen' # or 'gen'?
另一个例子是将显式的for..in yield
结构重构为yield from
表达式。考虑以下代码:
def outer_gen():
var.set('outer_gen')
for i in gen():
yield i
assert var.get() == 'outer_gen'
我们希望将其重构为使用yield from
。
def outer_gen():
var.set('outer_gen')
yield from gen()
assert var.get() == 'outer_gen' # or 'gen'?
以上示例说明,当使用yield from
重构生成器代码时,如果可能导致上下文更改泄漏,则是不安全的。
因此,唯一定义明确且一致的行为是**始终**隔离生成器中的上下文更改,无论它们如何被迭代。
PyThreadState_GetDict()
应该使用执行上下文吗?
不会。PyThreadState_GetDict
基于TLS,更改其语义将破坏向后兼容性。
PEP 521
PEP 521提出了一种解决该问题的替代方案,该方案通过两种新方法扩展了上下文管理器协议:__suspend__()
和__resume__()
。类似地,异步上下文管理器协议也通过__asuspend__()
和__aresume__()
进行扩展。
这允许实现管理非局部状态的上下文管理器,这些管理器在生成器和协程中表现正确。
例如,考虑以下使用执行状态的上下文管理器:
class Context:
def __init__(self):
self.var = contextvars.ContextVar('var')
def __enter__(self):
self.old_x = self.var.get()
self.var.set('something')
def __exit__(self, *err):
self.var.set(self.old_x)
使用PEP 521的等效实现:
local = threading.local()
class Context:
def __enter__(self):
self.old_x = getattr(local, 'x', None)
local.x = 'something'
def __suspend__(self):
local.x = self.old_x
def __resume__(self):
local.x = 'something'
def __exit__(self, *err):
local.x = self.old_x
这种方法的缺点是增加了上下文管理器协议和解释器实现的复杂性。这种方法也可能对生成器和协程的性能产生负面影响。
此外,PEP 521中的解决方案仅限于上下文管理器,并且没有提供任何机制来在异步任务和回调中传播状态。
是否可以在不修改CPython的情况下实现执行上下文?
不会。
确实,可以为协程在库中实现“任务本地”的概念(例如,参见[29]和[30])。另一方面,生成器由Python解释器直接管理,因此它们的上下文也必须由解释器管理。
此外,执行上下文根本无法在第三方模块中实现,否则标准库(包括decimal
)将无法依赖它。
我们是否应该更新sys.displayhook和其他API以使用EC?
诸如通过覆盖sys.stdout
重定向stdout或通过覆盖sys.displayhook
函数指定新的异常显示挂钩之类的API,**旨在**影响整个Python进程。它们的使用者假设更改它们的效果将在所有操作系统线程中可见。因此,我们不能仅仅让这些API使用新的执行上下文。
也就是说,我们认为可以设计新的上下文感知API,但这超出了此PEP的范围。
Greenlets
Greenlet是Python协作调度的替代实现。虽然greenlet包不是CPython的一部分,但像gevent这样的流行框架依赖于它,并且重要的是greenlet可以被修改以支持执行上下文。
从概念上讲,greenlet的行为与生成器非常相似,这意味着可以在greenlet进入和退出周围进行类似的更改以添加对执行上下文的支持。此PEP提供了执行此操作所需的必要C API。
上下文管理器作为修改的接口
此PEP专注于启用执行上下文的基本操作的底层机制和最小API。
为了开发人员的方便,可以在contextvars
模块中添加高级上下文管理器接口。例如:
with contextvars.set_var(var, 'foo'):
# ...
设置和恢复上下文变量
ContextVar.delete()
方法从最顶层的逻辑上下文中删除上下文变量。
如果在最顶层的逻辑上下文中找不到该变量,则会引发 LookupError
,类似于当 var
不在作用域内时,del var
引发 NameError
。
当(很少)需要正确恢复逻辑上下文的状态时,此方法很有用,例如,当嵌套生成器想要临时修改逻辑上下文时。
var = contextvars.ContextVar('var')
def gen():
with some_var_context_manager('gen'):
# EC = [{var: 'main'}, {var: 'gen'}]
assert var.get() == 'gen'
yield
# EC = [{var: 'main modified'}, {}]
assert var.get() == 'main modified'
yield
def main():
var.set('main')
g = gen()
next(g)
var.set('main modified')
next(g)
上面的示例只有在 gen()
中有一种方法可以从逻辑上下文中删除 var
时才能正常工作。在 __exit__()
中将其设置为“先前值”将掩盖在迭代之间在 main()
中进行的更改。
ContextVar API 的替代设计
具有堆叠值的逻辑上下文
根据本 PEP 中提出的设计,逻辑上下文是一个简单的 LC({ContextVar: value, ...})
映射。另一种表示方法是为每个上下文变量存储一个值栈:LC({ContextVar: [val1, val2, ...], ...})
。
然后,ContextVar
方法将是
get(*, default=None)
– 遍历逻辑上下文栈,并从第一个非空逻辑上下文返回顶部值;push(val)
– 将val 推送到当前逻辑上下文的值栈中;pop()
– 从当前逻辑上下文的值栈中弹出顶部值。
与使用 set()
和 delete()
方法的单值设计相比,基于栈的方法允许更简单地实现设置/恢复模式。但是,这种方法的心理负担被认为更高,因为需要考虑两个栈:LC 栈和每个 LC 中的值栈。
(这个想法是由 Nathaniel Smith 提出的。)
ContextVar“设置/重置”
另一种方法是从 ContextVar.set()
返回一个特殊对象,它将表示在当前逻辑上下文中对上下文变量的修改。
var = contextvars.ContextVar('var')
def foo():
mod = var.set('spam')
# ... perform work
mod.reset() # Reset the value of var to the original value
# or remove it from the context.
这种方法的关键缺陷在于,可以将上下文变量“修改对象”传递给在不同执行上下文中运行的代码,这会导致未定义的副作用。
向后兼容性
此提案保留了 100% 的向后兼容性。
被拒绝的想法
复制threading.local()接口
考虑并拒绝为上下文变量选择类似 threading.local()
的接口,原因如下
- 对标准库和 Django 的调查表明,绝大多数
threading.local()
使用都涉及单个属性,这表明命名空间方法在该领域并不那么有用。 - 使用
__getattr__()
而不是.get()
进行值查找,无法指定查找的深度(即仅搜索最顶层的逻辑上下文)。 - 单值
ContextVar
在可见性方面更容易推理。假设ContextVar()
是一个命名空间,并考虑以下情况ns = contextvars.ContextVar('ns') def gen(): ns.a = 2 yield assert ns.b == 'bar' # ?? def main(): ns.a = 1 ns.b = 'foo' g = gen() next(g) # should not see the ns.a modification in gen() assert ns.a == 1 # but should gen() see the ns.b modification made here? ns.b = 'bar' yield
以上示例表明,推理同一上下文变量的不同属性的可见性并非易事。
- 单值
ContextVar
允许直接实现查找缓存; - 单值
ContextVar
接口允许 C-API 简单且与 Python API 基本相同。
协程默认不泄漏上下文更改
在本 PEP 的 V4(版本历史)中,协程被认为在执行上下文中与生成器完全相同:在等待的协程中发生的更改在外层协程中不可见。
这个想法被拒绝的理由是,它破坏了任务和线程模型之间的语义相似性,更具体地说,它使得无法可靠地实现修改上下文变量的异步上下文管理器,因为 __aenter__
是一个协程。
附录:HAMT 性能分析
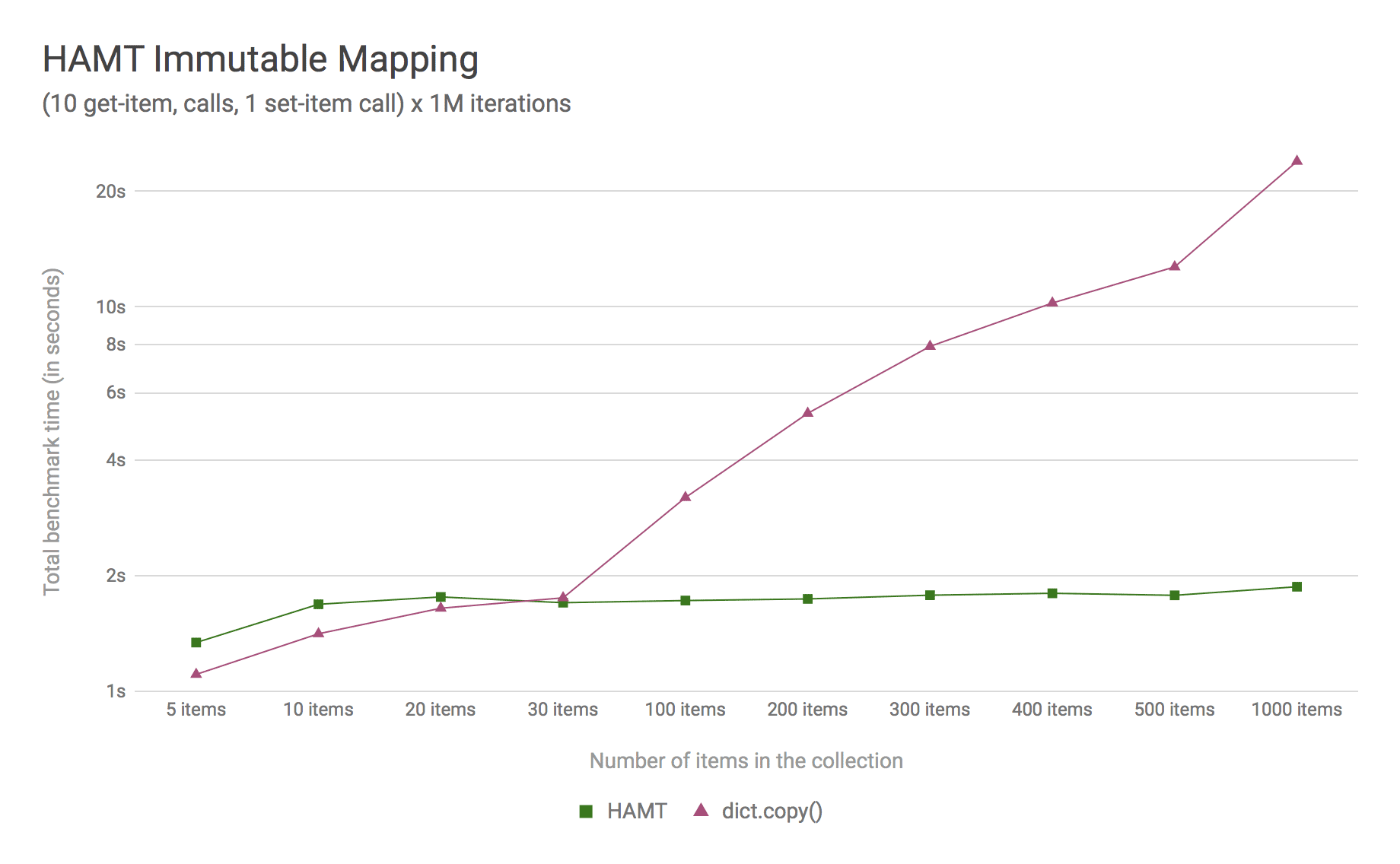
图 1. 基准代码可以在这里找到:[9]。
上图表明
- HAMT 对所有基准测试的字典大小显示接近 O(1) 的性能。
dict.copy()
在大约 100 个项目时变得非常慢。
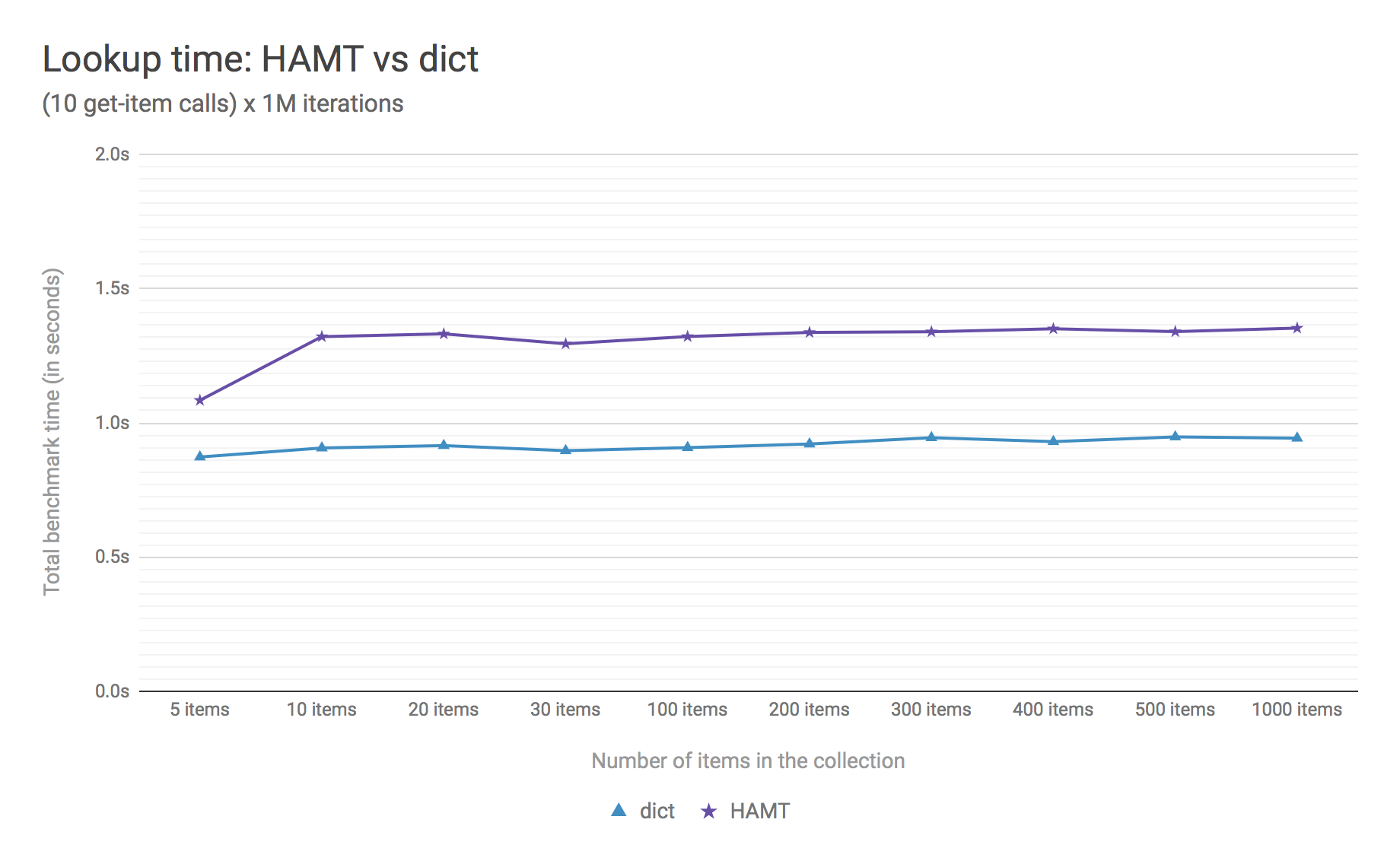
图 2. 基准代码可以在这里找到:[10]。
图 2 比较了 dict
与基于 HAMT 的不可变映射的查找成本。HAMT 查找时间平均比 Python dict 查找慢 30-40%,这是一个非常好的结果,考虑到后者已经过非常好的优化。
有一些研究 [8] 表明 HAMT 的性能还有进一步改进的可能。
CPython 的 HAMT 参考实现可以在这里找到:[7]。
致谢
感谢 Victor Petrovykh 在该主题周围进行了无数的讨论,以及 PEP 校对和编辑。
感谢 Nathaniel Smith 提出 ContextVar
设计 [17] [18],推动 PEP 朝着更完整的设计发展,并提出了在线程状态中使用上下文栈的想法。
感谢 Alyssa (Nick) Coghlan 在邮件列表中提出的众多建议和想法,以及提出了导致最初 PEP 版本完全重写的情况 [19]。
版本历史
- 初始修订版,发布于 2017 年 8 月 11 日 [20]。
- V2 发布于 2017 年 8 月 15 日 [21]。
导致第一个版本完全重新设计的根本限制是,无法实现一个迭代器,它将以与生成器相同的方式与 EC 交互(参见 [19])。
版本 2 是一个完整的重写,引入了新的术语(本地上下文、执行上下文、上下文项)和新的 API。
- V3 发布于 2017 年 8 月 18 日 [22]。
更新
- V4 发布于 2017 年 8 月 25 日 [31]。
- 规范部分已完全重写。
- 协程现在有自己的逻辑上下文。这意味着协程、生成器和异步生成器在与执行上下文的交互方面没有区别。
- 上下文键重命名为上下文变量。
- 删除了生成器和协程在逻辑上下文隔离方面的区别。
- V5 发布于 2017 年 9 月 1 日:当前版本。
- 协程默认情况下没有逻辑上下文(恢复为 V3 语义)。在 协程默认情况下不泄漏上下文更改 部分阅读有关动机的更多信息。
高级规范 部分也进行了更新(特别是生成器和协程子部分)。
- 所有 API 都已放置到
contextvars
模块中,工厂函数已更改为类构造函数(ContextVar
、ExecutionContext
和LogicalContext
)。感谢 Alyssa 提供的想法 [33]。 ContextVar.lookup()
已重命名回ContextVar.get()
并获得了topmost
和default
关键字参数。添加了ContextVar.delete()
。请参阅 Guido 在 [32] 中的评论。
- 新的
ExecutionContext.vars()
方法。在 枚举上下文变量 部分阅读有关它的信息。 - 修复了
ContextVar.get()
缓存错误(感谢 Nathaniel!)。 - 新的 被拒绝的想法、“yield from” 应该泄漏上下文更改吗?、ContextVar API 的替代设计、设置和恢复上下文变量 和 上下文管理器作为修改的接口 部分。
- 协程默认情况下没有逻辑上下文(恢复为 V3 语义)。在 协程默认情况下不泄漏上下文更改 部分阅读有关动机的更多信息。
参考文献
版权
本文档已置于公共领域。
来源:https://github.com/python/peps/blob/main/peps/pep-0550.rst
上次修改时间:2023-10-11 12:05:51 GMT